StemForAll
2025
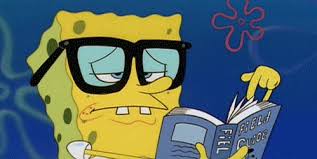
Organizers:
Alex Iosevich (University of Rochester) and Azita Mayeli
(CUNY)
Registration
Form
Registration Deadline: May 10, 2025
Last update: Saturday, May 4, 2025
Program dates: July 28, 2025 - August 8, 2025
Introduction: Welcome to StemForAll2025 summer workshop. All
the interested Rochester area students are welcome to participate.
The registration process is only used to assign the students to
suitable projects. The main idea behind the workshop is to share the
research we are doing with undergraduate students for the purpose of
familiarizing them with research methods and techniques. Quite often
research papers result from these discussions, but the main emphasis
is on learning and the creative process. In 2025, the program in
Rochester will be organized by Alex Iosevich (UR), Steven
Kleene (UR). and Azita Mayeli (CUNY).
Program instructors: Gio Garza (University of Delaware), Dan
Geba (UR), Nadia
Lab Hab, Gabe
Hart (UR), Alex Iosevich (UR), Stephen Kleene (UR), Tran Duy Anh Le
(UCSD), Caleb Marshall (UBC), Azita Mayeli (CUNY), Alice Quillen
(UR), Curt Signorino (UR), Nathan Skerrett (UR), Kunxu
Song (University of
Kansas), Zachary Tan
(UR).
Program
Participants:
The participants are
listed under the
various projects
below, but you can
also get this
information by
clicking here.
History of the program: StemForAll has been running at the
University of Rochester since 2018. In one form or another this
program has existed at the University of Rochester and University of
Missouri since 2001. Many of its participant have since obtained
Ph.Ds in mathematics and related fields and have become successful
researchers. The links to the previous programs can be found here.
Expansion: The StemForAll program will be expanding in 2026.
In addition to the annual program we have been running in the
Rochester area for several years, analogous programs are going to
run at the following institutions:
Missouri State University (Steven Senger)
Virgina Tech (Eyvindur Palsson)
Ohio State University (Krystal Taylor)
The StemForAll Team consists mathematicians who have committed to
running a two-week StemForAll undergraduate research program at
their home institutions during Summer 2025. The members of the team
are going to create a joint database of research problems and other
research materials, and share those with all the other affiliates.
Rochester StemForAll location and time: StemForAll2025 in the
Rochester area is going to take place in July/August 2025 in the
Hylan Building on the University of Rochester campus. Most of the
groups are going to work on the 11th floor, but we may need other
space as well.
Structure of the workshop: StemForAll2025 is going to consist
of a wide variety of projects, listed below, involving the
interaction of pure and applied mathematics, statistics, physics and
data science. Many of the projects are strongly related, which
should lead to a considerable amount of interaction. We are going to
have a blast!
Program Schedule
Workshop Projects:
Signal recovery themed projects:
i) Exact
Signal Recovery
Project
supervisors: Gio Garza, Alex
Iosevich, Tran Duy Anh Le, and Azita Mayeli
Research
meeting location: Hylan 909
Project
description: Suppose that a signal of length N is
transmitted via its discrete Fourier transform and some of the
signal is lost in the transmission due to noise or interference.
Under what conditions is it possible
to recover the original signal exactly? This
innocent looking question quickly leads to some interesting
techniques and ideas from analysis, combinatorics and other
areas of mathematics. We are going to investigate these types of
questions from both the theoretical and computational points of
view.
Project
participants: (13) Jenna Ahn
(jahn14@u.rochester.edy), Bukhari Fandi
(buxariom@gmail.com), Yujia Hu
(yhu77@u.rochester.edu), Joshua Iosevich
(joshuaiosevich@gmail.com), Julian King
( jhk2@geneseo.edu), Alhussein
Khalil (akhalil3@u.rochester.edu), Tran
Duy Anh Le (duyanh10t1hsgs@gmail.com),
Lyudovik
Spencer
(slyudovy@u.rochester.edu),
Kelvin Nguyen (knguy43@u.rochester.edu),
Nathaniel
Shaffer
(nshaffe4@u.rochester.edu),
Claire Strobel
(cstrobel@u.rochester.edu),
Marina
Tilgadas
(mtilgad@u.rochester.edu),
Mingyu
Zhang (mzhang95@u.rochester.edu),
Showmee Zhou (zzhou69@u.rochester.edu)
Things to learn before the workshop:
Basic Fourier analysis in
Perhaps the most direct way to do this
that also introduces you to the subject
matter of this research group is to
watch the youtube videos of my lectures
at Hausdorff Institute of Mathematics in
Bonn: Lecture
1, Lecture
2, Lecture
3, Lecture
4.
Things to read: After watching
the lectures above, I recommend reading
honors theses by William Hagerstrom, Tran Duy Anh Le, and
Isaac Li. You can find them on the
UR honors thesis website
here. We also recommend the
following papers that can be found
on arxiv.org: arXiv:2504.14702,
arXiv:2502.13786, arXiv:2411.19195,
arXiv:2311.04331. Please note that
different papers may have different
normalizations for the Fourier
transform. Such is life!
ii) Fourier Analysis and Recovery of Missing
Values in Times Series
Project supervisors: Will Burstein, Alex
Iosevich, Azita Mayeli, and Hari Nathan
Research meeting location:
Hylan 909
Project
description: In a paper in preparation, the project
supervisors showed that the
performance of virtually any reasonable time series
forecasting engine can be improved, with high
probability, by judiciously
filtering out a certain number of
small Fourier coefficients at the end of the forecast.
The purpose of this project is to optimize and streamline this
process. We will also make an effort to unify our approach with the
classical techniques of exact signal recovery that will be explored
by the Exact Signal Recovery research group.
Project
participants: (8) William Du
(jdu14@u.rochester.edu), Joshua Iosevich
(joshuaiosevich@gmail.com), Tran Duy Anh Le
(duyanh10t1hsgs@gmail.com), Kaifeng Lu
(klu15@u.rochester.edu), Qianxiang Shen
(qshen11@u.rochester.edu), Oliver Shevick
(shevick.oliver@gmail.com), Kunwar Arpid
Singh (kunwar22@iiserb.ac.in)
Things
to learn
before the
workshop: Basic
Fourier analysis
in
Perhaps the most
direct way to do
this that also
introduces you
to the subject
matter of this
research group
is to watch the
youtube videos
of my lectures
at Hausdorff
Institute of
Mathematics in
Bonn: Lecture 1,
Lecture 2, Lecture 3, Lecture 4.
Things to
read: For
the moment,
please take a
look at the
slides of Alex
Iosevich's
colloquium talk
at Northwestern
University here.
iii)
Sampling on Manifolds and
Fourier Uncertainty
Principle
Project supervisors: Alex
Iosevich, Azita Mayeli, and
Steven Kleene
Research
meeting location:
Math Lounge,
9th floor of Hylan
Bldg.
Project
description: In
a paper in preparation, Iosevich,
Renfrew and Wyman
are studying the
problem of how
many random
samples are
needed to
reconstruct a
band-limited
function on a
compact
Riemannian
manifold without
a boundary.
They are studying
the stability of the
recovery process in
terms of the
smallest singular
values of the
underlying matrix.
In this project, we
are going to conduct
extensive numerical
experiments designed
to get a feel for
this process on
concrete Riemannian
manifolds. We are
also going to study
the Fourier
uncertainty
principle on
Riemannian
manifolds, following
up on a recent paper
on this topic by
Iosevich, Mayeli and
Wyman. We are also
going to explore
various applied and
computational
aspects of these
problems.
Project
participants:
(3) Nikash Gajate
(ngajate@u.rochester.edu),
Zekuan Guo
(zguo26@u.rochester.edu),
Kelvin
Nguyen
(knguy43@u.rochester.edu)
Things to learn
before the
workshop: For
now, please read
Wolff's notes on
harmonic analysis here. There may be
things in there that
require background
you do not have. No
worries! Just keep
reading and looking
things up. This is
research!
Things to read: Please
take a look at the
following paper on
arxiv.org:
arXiv:2411.09057
Machine
Learning themed projects:
i) Election Forecasts and Neural Networks
Project supervisors: Alex Iosevich and Hari Nathan
Research meeting location:
11 floor of Hylan Bldg.
Project description: We are going to examine the
polling data preceding the November 5, 2024 Presidential
Election and design neural
network models to forecast the outcome in
terms of the popular vote, the number of electoral votes
and the outcome of the election. We shall then compare
the performance of our models against the actual outcome
of the elections.
Project
participants: (6)
Anastasia
Chyzh
(Achyzh@u.rochester.edu),
Ji
Woong Hong
(jhong36@u.rochester.edu),
Asilbek
Ismatilloev
(aismatil@u.rochester.edu),
Naomi Kim
(nkim31@u.rochester.edu),
David Yen
(dyen3@u.rochester.edu)
ii)
Sales
modeling with
economic
indicators
Project
supervisors: Gabe
Hart, Alex
Iosevich and
Steven Senger
Research
meeting
location: 11th
floor Hylan Bldg.
Project
description:
We are going
to build and
test neural
network models
with economic
indicator
regressors
to
effectively
predict future
sales in
retail. A
variety of
neural network
models will be
built using
tensorflow,
keras,
facebook
prophet and
others. The
second major
part of this
project is
using generative
AI
for parameter
tuning and
inventory
optimization.
Theoretical
aspects of
this problem
will be
considered as
well.
Connections
with the
theory of
exact signal
recovery will
also be
explored.
Project
participants:
(19)
Liam Hillis
(lhillis@u.rochester.edu),
Ji Woong Hong
(jhong36@u.rochester.edu),
Jingwen Hu
(jhu62@u.rochester.edu),